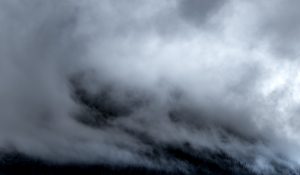
Archive of posts tagged: Machine Learning
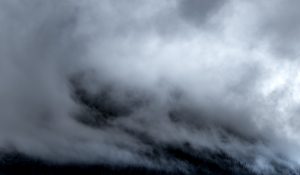
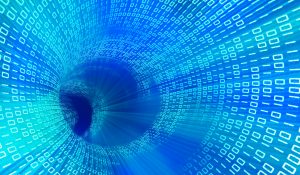
The New Bottlenecks of ML Training: A Storage Perspective
Machine Learning (ML), specifically Deep Neural Networks (DNNs), is stressing storage systems in new ways, moving the training bottleneck to the data ingestion phase, rather than the actual learning phase. Training these models is data-hungry, resource-intensive, and...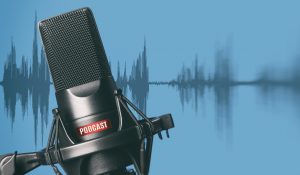
Catalyzing Computing Podcast – Computer Architecture with Mark D. Hill
[Editor’s Note: This article originally appeared on the CCC blog (part 1 and part 2) and is re-posted here with permission.] A new episode of the Computing Community Consortium‘s (CCC) official podcast, Catalyzing Computing, is now available. In this episode, Khari...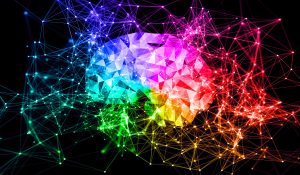
Nanoscale Optoelectronic AI Processing
In a previous blog post, we summarized some advances in optical computing that enable the implementation of low-energy optical-convolutional layers using phase masks and angle-sensitive pixels. Such approaches also present multiple challenges, such as lack of...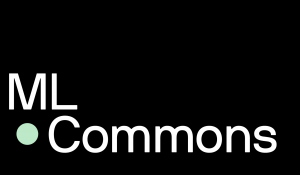